BMC Medicine volume 21, Article number: 466 (2023 ) Cite this article
Each mother–child dyad represents a unique combination of genetic and environmental factors. This constellation of variables impacts the expression of countless genes. Numerous studies have uncovered changes in DNA methylation (DNAm), a form of epigenetic regulation, in offspring related to maternal risk factors. How these changes work together to link maternal-child risks to childhood cardiometabolic and neurocognitive traits remains unknown. This question is a key research priority as such traits predispose to future non-communicable diseases (NCDs). We propose viewing risk and the genome through a multidimensional lens to identify common DNAm patterns shared among diverse risk profiles. Rna Sequencing

We identified multifactorial Maternal Risk Profiles (MRPs) generated from population-based data (n = 15,454, Avon Longitudinal Study of Parents and Children (ALSPAC)). Using cord blood HumanMethylation450 BeadChip data, we identified genome-wide patterns of DNAm that co-vary with these MRPs. We tested the prospective relation of these DNAm patterns (n = 914) to future outcomes using decision tree analysis. We then tested the reproducibility of these patterns in (1) DNAm data at age 7 and 17 years within the same cohort (n = 973 and 974, respectively) and (2) cord DNAm in an independent cohort, the Generation R Study (n = 686).
We identified twenty MRP-related DNAm patterns at birth in ALSPAC. Four were prospectively related to cardiometabolic and/or neurocognitive childhood outcomes. These patterns were replicated in DNAm data from blood collected at later ages. Three of these patterns were externally validated in cord DNAm data in Generation R. Compared to previous literature, DNAm patterns exhibited novel spatial distribution across the genome that intersects with chromatin functional and tissue-specific signatures.
To our knowledge, we are the first to leverage multifactorial population-wide data to detect patterns of variability in DNAm. This context-based approach decreases biases stemming from overreliance on specific samples or variables. We discovered molecular patterns demonstrating prospective and replicable relations to complex traits. Moreover, results suggest that patterns harbour a genome-wide organisation specific to chromatin regulation and target tissues. These preliminary findings warrant further investigation to better reflect the reality of human context in molecular studies of NCDs.
Identifying the impact of maternal risk factors on foetal health and child outcomes is challenging because each mother–child dyad represents a unique combination of genetic, environmental, and random chance effects. This complexity accounts for why identical twins demonstrate differences in health outcomes or why foetal alcohol exposure can have a broad spectrum of features rather than a single phenotype. Moreover, the range and complexity of maternal risk factors that impact the foetus go beyond direct in utero effects and can include intergenerational effects [1].
Regardless of the sources or types of risk, research suggests that such multifactorial risk exposures set off a ripple effect across the human genome, impacting the regulation of a multitude of biological pathways [2]. It is believed that risk exposure leads to disease in offspring due to “foetal programming”, a concept arising from the developmental origins of disease hypothesis (DOHaD) [3]. Epigenetic modifications of the genome are mechanisms through which risk exposure may dysregulate foetal programming [4, 5]. These non-genetic molecular changes to the genome modify the DNA’s physical 3D organisation and its interaction with nuclear machinery [6, 7]. The readiness of genes to be expressed is thus altered. Epigenetic modifications can work together to change the gene expression patterns of the entire cell. The best-studied epigenetic mechanism in humans is DNA methylation (DNAm). DNAm describes the addition of a methyl group to a cytosine base located upstream of a guanine base in a DNA sequence, which together is known as a CpG site.
Most research attempts to pinpoint single or neighbouring clusters of CpG sites associated with a given risk or outcome, such as smoking or birth weight. Researchers now venture to connect these points across multiple sites on different chromosomes. For instance, Elliott and colleagues (2014) generated a smoking methylation score in adults that combined 183 CpG sites to distinguish never and former smokers from current smokers. This methylation score separated these two categories with 100% sensitivity and 97% specificity in a male population of European descent. When multiple sites are linked, theoretical and empirical evidence suggests that DNAm can provide insight into an individual’s past experiences.
Epigenetic analyses that focus on discrete risk exposures and molecular sites create models that are easier to understand and analyse, but these models do not reflect the clinical reality of non-communicable diseases (NCDs). Each person experiences a unique array of risks whose genomic targets are interactive and multidimensional in nature. Hence, we propose to view risk exposure and the genome as interconnected parts of an engine that drives the trajectory of health. This proof-of-concept study tests this idea of integrating context into statistical models using basic multidimensional methods.
To begin, we asked how to address the challenge of examining the phenomenon of unique risk exposures in each individual across a population. We decided to develop a maternal risk profile (MRP) centred around the maternal behaviour of smoking while pregnant. This health behaviour is a telling marker of stress among physical, mental, and social determinants of disease [8]. Smoking during pregnancy is often linked to other lifestyle risk factors (e.g. drug and/or alcohol abuse) and stressors (e.g. lower socioeconomic status [9]). It also has known direct effects on the foetal environment and physiology [10]. Lastly, it is a common foetal exposure linked to a broad range of NCDs in later life [11,12,13,14,15,16]. By anchoring foetal risk to the multifactorial phenomenon of maternal smoking, we hope to capture a risk profile that intersects health domains as would occur in clinical reality.
From a physiological perspective, exposure to MRP may behave like other stresses and have a normative range within the general population. Therefore, we used multiple variables related to maternal smoking in previous literature to generate a population-based MRP. This reduces the chance of drawing conclusions that only pertain to a subset of the population.
Next, we considered how to view the molecular components. A multivariate model of associated DNAm changes may be particularly applicable in the study of NCDs. These diseases are etiologically distinct from processes like cancers that typically can be traced to single molecular events. NCDs tend to arise from many small and dynamic cellular changes that lead to an overall shift in the cellular and physiological state of the whole person. These multiple small changes reflect the interplay of genes, environment, and random chance.
We theorise that coordinated shifts in genome-wide DNAm (gwDNAm) could be the common mechanistic link between individuals who encounter different maternal influences yet share similar phenotypic traits. We tested this by seeking recurring patterns of gwDNAm in cord blood that relate to population-based MRPs. In this way, gwDNAm patterns represent population-normalised molecular signatures of risk exposure. We investigated how these gwDNAm patterns may relate to the development of NCDs by observing their relation to anthropometric and neurodevelopmental child outcomes. We repeated this analysis over time as children aged and in an independent cohort. We outline our analysis workflow in Fig. 1.
Analysis workflow schematic. Part 1 (aqua) involves component-based analysis to integrate two multidimensional data sets, risk and DNAm. We obtain a new set of variables representing variability in methylation at birth that reflect risk exposure, which we call genome-wide DNAm patterns (gwDNAm patterns). Part 2 (purple) involves observing the interaction of variables and covariates that could lead to a given outcome using decision tree analysis. This is directly compared to relations to outcomes that could arise through random chance alone. In this way, this analysis can inform us of robust relations between heterogeneous risks and complex traits
Model discovery was conducted in the population-based ALSPAC longitudinal cohort. This initial cohort collected data on 14,541 pregnancies and 14,676 foetuses in the former county of Avon, United Kingdom (UK) with expected delivery dates from 1 April 1991 to 31 December 1992. This cohort has been extensively described [17, 18] with specific details regarding variables pertinent to our analysis available in Additional file 1.
To develop a multidimensional risk profile from the viewpoint of foetal programming, we apply a context-based approach that uses population-based data to integrate multiple MRP-related variables. The normative values for blood pressure in children exist as continuous ranges that are based on sex, age, and height percentiles. Similarly, we regard to foetal risk exposure as a continuous and interdependent variable. The risk factors traverse gestational (e.g. maternal smoking in pregnancy, pregnancy factors, foetal health and growth, etc.), family (e.g. smoking in the father, grandparents, other household members, etc.), and social effects (e.g. education, vocation, etc.). These data were gathered from maternal self-reports and linked clinical records.
We selected outcomes based on their relevance to maternal smoking exposure (Table 1) as identified in previous literature [14, 19,20,21]. All cardiometabolic outcome values were converted to internal z-scores (sex-specific) using data from the entire ALSPAC cohort unless otherwise specified. To control for the impact of early weight and length accrual on later life physical outcomes, individual infant growth trajectories calculated for this cohort using multilevel modelling were included in relevant models [22]. At age 18, study children were sent “fair processing” materials describing ALSPAC’s intended use of their health and administrative records, and they were given clear means to consent or object via a written form. Data were not extracted for participants who objected or who were not sent fair processing materials.
ARIES is a sub-study of ALSPAC child-mother pairs with available DNAm data. Blood samples were collected at three time points: birth (cord blood) and at ages 7 and 17 years [24]. At later ages, DNA was extracted from either the buffy coat or whole blood samples. DNAm data measurements were performed using the Illumina Infinium HumanMethylation450 BeadChip (450K BeadChip). The technical details of data normalisation, filtering unreliable or low variance probes, and managing batch effect, blood cell type composition, and confounders are available in Additional file 1. This left 185,466 CpG sites remaining. DNAm beta values were used in all analyses.
The Generation R Study (GenR) is a population-based prospective pregnancy cohort study. It included 9778 women and their children born between April 2002 and January 2006. We used cord blood 450K BeadChip DNAm data from a subgroup consisting of 969 children of European descent described in detail [25]. In total, 686 children had complete data for these analyses. Besides detailed pregnancy data, this cohort collected substantial offspring data, including anthropometric data at birth, in infancy, and at ages 6, 10, and 13 years.
In part one of the study (see Fig. 1, aqua), we needed to link the multifactorial effect of risk to thousands of DNAm sites across the genome. We employed component-based analysis, a widely used technique to analyse “big data” where the number of subjects is far exceeded by the number of data points. Component analysis makes “big data” more manageable by representing numerous variables using a far smaller number of variables, usually 100- to 1000-fold fewer. How variables select a single variable to represent them varies, but it remains that using fewer representative variables can increase study power. Also, it can attenuate false-positive results driven by the reliance on single points of data which can suffer from known and unknown sources of error and bias [26]. Bias arising from genetic ancestry- or cohort-specific characteristics at individual sites of DNAm is particularly challenging in this field [5]. Component-based analysis also allowed us to leverage population-based data to assign a unique risk profile to each child that is “normalised” in the context of the whole population. In other words, the MRP positions each child relative to other children.
Much like the clinical observation of a patient, component-based analysis enables us to view prenatal risk exposure as multifactorial and existing on a continuous normal distribution. Since we link gwDNAm patterns to MRPs, inferences about DNAm are made relative to the whole population. This diminishes the risk of drawing statistically flashy results that only exist in the subset of individuals with DNAm data.
In part two of the study (Fig. 1, purple), we aim to model the reality of complex interactions between multiple biological inputs that lead to a given phenotype. We used decision tree-based analysis, a family of methods that gained popularity in medicine during the COVID-19 epidemic as a resource allocation tool to identify patients most likely to suffer serious morbidity or mortality. Decision tree-based methods complement a clinician’s intuitive integration of numerous patient features to draw a clinical conclusion. The goal is to discover patient features that are relevant to the patient’s outcome, regardless of their effect size. The decision tree structure enables it to detect non-linear and complex interactive effects that may reveal novel yet relevant relations. This ability helps to account for “atypical” individuals, where a feature that typically predicts one outcome is modified by other patient characteristics and leads to an alternate outcome.
Random forests (RFs) are a type of decision tree analysis that generates thousands of trees through an algorithm that randomly shuffles data values. The collation of these results is akin to the consensus of thousands of doctors who have each encountered thousands of unique patients. This inherent property of RF diminishes overfitting to a specific subset of a population. Further details on Parts 1 and 2 of our analysis are discussed below and in Additional file 1. All statistical analyses were performed using R software (version 4.0.0) [27]. Multiple testing corrections and power calculations are unsuitable for this study design since we are not conducting multiple independent tests or seeking differences between groups.
We used component-based analysis to extract MRP-related DNAm patterns in three steps. We summarise these steps (1-A, 1-B, and 1-C) below.
We used the Factor Analysis for Mixed Data (FAMD) method, a principal component-based technique, as described in the FactoMineR R-package [28]. We refer to these mathematical representations of MRPs as “dimensions”, as the package authors did. We performed model-based missing value imputation with the built-in imputeFAMD function. The best-fit model included eight variables: birth weight, maternal smoking during pregnancy, household smoking, maternal grandfather’s history of smoking, maternal partner’s smoking history, maternal grandmother’s history of smoking, maternal history of smoking, and grandmother’s smoking while pregnant with mother, hereafter referred to as MRP-related variables (Table 2). The details regarding sampling adequacy, variance captured, and variable contributions are available in Additional file 1.
The MRPs served as “bait” to capture relevant DNAm patterns in cord blood using the sPLS function in the sgPLS R-package. The objective of PLS modelling is to predict MRP with DNAm data (depicted as Y and X, Additional file 2, Figure S1, inset). The PLS output represents sources of variability within the DNAm data using a small number of components that best represent MRPs. Components are extracted such that they are uncorrelated. In this paper, we refer to “components” as the mathematical representation of the molecular variation we refer to as gwDNAm patterns.
The PLS weightings among CpG sites trained from our cord blood model were the “template” to test the same patterns in different DNAm data sets while blinded to their corresponding MRP data. We used the predict function of the sgPLS package to test the cord blood model on peripheral blood at ages 7 and 17 years in ARIES and perform external validation using cord blood from GenR.
The RF method can accommodate two important considerations: first, non-normal residual distributions found in omic and risk exposure data that violate traditional statistical assumptions and, second, indirect and/or non-linear relations between predictors and outcomes. RF uses an ensemble of decision trees derived from bootstrapped samples of the data set and random variable selection to optimise model stability and attenuate overfitting [29]. RF ranks the relevance of competing variables in predicting outcomes by assigning an importance score (calculated as the mean decrease in accuracy) [29]. Alluding to our earlier analogy about decision tree analysis, suppose those thousands of doctors “rated” how relevant a variable was to predict an outcome. The importance score represents a collation of these ratings, with a higher score representing a variable that was more helpful in deciding the outcome.
To ensure the robust selection of variables, we empirically evaluate what threshold of importance is considered relevant to predicting an outcome using a two-step RF strategy, [30] which is described briefly below.
We used the Boruta algorithm (from R-package of the same name) to select all variables relevant to an outcome [31]. This is well-suited to our study, which aims to understand the mechanisms underlying a process. Instead of using prediction metrics, Boruta selects relevant variables by testing if their relation to the outcome exceeds that which could occur by chance. This is performed by generating shadow variables, which are shuffled copies of real variables such that their values no longer have any correlation with the outcome. Using a binomial test (p < 0.05), the model can estimate the likelihood a real variable will exceed the importance of a shadow variable. Thus, shadow variables introduce additional randomness to the model, making it more robust to random chance or correlations that can lead to false positives. We included the following previously associated covariates in all models: sex, maternal educational level, paternal social class, and in models using DNAm data, blood cell type proportions [32,33,34].
We calculated model metrics using the caret R-package [35]. We calculated the performance metrics of the model using Boruta-selected variables (implemented in the caret R-package, [35] ntree = 500). We conducted re-sampling using fivefold cross-validation with 3 repeats and considered a combination of model performance metrics, namely mean squared error (MSE), mean absolute error, and coefficient of determination (R2). In this way, we compared the predictive performance of models using DNAm data, MRPs, and MRP-related variables. We further tested the performance of models using DNAm data from peripheral blood and GenR cord blood.
All models included sex, maternal education, and paternal social status as covariates. We selected the latter two variables among other social confounders after evaluation of multicollinearity and data loss due to missingness (see Additional file 1: Evaluating confounder effects). To understand the impact of confounders specifically on gwDNAm, we also performed an additional analysis using another component-based technique, singular value decomposition (SVD). SVD has been applied to identify covariation with confounding variables in DNAm data using R-package ChAMP [36]. Models of cardiometabolic outcomes included growth variables given the correlation between earlier and later time points in child developmental trajectories.
We performed enrichment-based analysis to evaluate the molecular relevance of gwDNAm patterns based on publicly available data as previously described (Additional file 1: Enrichment-based analysis—finding molecular relevance).
Among ALSPAC subjects, 15,454 newborns had at least one data item collected at birth. The construction of MRPs included data from these newborns and their mothers. DNAm in ARIES was measured from cord blood at birth and around ages 7 (mean 7.5, standard deviation (SD) 0.1) and 17 (mean 17.1, SD 1) years in 914, 973, and 974 children, respectively. Previous studies comparing the baseline characteristics of families in the ALSPAC versus the ARIES datasets found that the ARIES mothers were older, reported less maternal smoking, attained a higher educational level, and were more likely employed in non-manual labour [24, 37]. Characteristics of MRP-related variables are tabulated in Table 2.
We performed factor analysis to represent the MRP-related variables as a composite score (see the “Methods” section—Part 1, Step 1-A). Based on model metrics, the top five dimensions were selected (scree plot in Additional file 2, Figure S1). Each dimension is a mathematical representation of the MRPs and describes a varying degree of contribution from all constituent MRP-related variables (see Table 2). Thus, children in the entire ALSPAC data set were assigned a score for each dimension, giving each individual a unique five-part profile of risk exposure.
Each of the first two dimensions captured nearly 20% of total data variability. We can evaluate the risk represented by each dimension by viewing its most prominent contributory variables (Fig. 2). For example, Dimension 1 primarily captures the infant’s grandmaternal data, i.e. the grandmother’s smoking history and whether the grandmother smoked while pregnant with the mother. In contrast, Dimension 2 captures similar information for the infant’s mother.
Bar graph of the relative contribution of variables (x-axis) to maternal risk profile construction. While each risk profile has varying contributions from all eight risk-related variables, certain variables dominate the construction of a dimension. The bars represent the relative contribution of each variable to a given dimension. Contributions that exceed the grey vertical line (calculated as in [20]) belong to variables that are considered the most representative of a dimension (see Additional file 1). Colours show variables that exceed this threshold and will be used to refer to each dimension in subsequent figures
While we refer to the MRP representations as “dimensions”, we use the term “components” to refer to gwDNAm patterns generated by the PLS method (see the “Methods” section—Part 1, Step 1-B). Each component mathematically represents a consistent pattern of DNAm at specific genomic sites that are related to MRP variability among subjects. PLS does not seek to account for the most variability in data but the most shared variance between two sets of data. In this way, PLS assigns component scores to each person that indicates how alike or unlike his/her DNAm variability is to that given component pattern, which in turn is related to MRP. Thus, every subject has a unique identity of gwDNAm that is related to his/her risk exposure. In Additional file 3, we provide the full list of CpGs most representative of each gwDNAm pattern.
Inherent in the technical measurement of biology is the overlap of variability between the variable of interest and confounders (see the “Methods” section—Confounder analysis). To identify the degree of overlap, we evaluated the correlation between components with confounders (Additional file 2: Figures S2-S5). There was strong evidence of relation to sex with Components 1 to 3, 5, 6, and 10. None showed strong relations to maternal education or paternal social status. The strongest correlations to cell type proportions were between Components 1 and 3 with granulocytes and CD4 cells and Component 6 with nucleated red blood cells.
Next, we show the relation between MRPs and gwDNAm patterns in Fig. 3. We observed that Dimension 1 (grandmaternal smoking features) correlated most with the DNAm patterns represented by Component 9. Dimension 2 (maternal smoking features) was most related to Component 14. Similarly, we observed the relation between Dimension 3 (smoking in mother/father/grandfather/other household members and infant birth weight) to Component 8, Dimension 4 (maternal smoking and birth weight) to Component 7, and Dimension 5 (smoking in mother/other household members and birth weight) to Components 13, 18, and 19. For the sake of brevity and interpretability, we will focus on these components and exclude discussion of confounder-related components (Components 1–6 and 10) in the “Results” section moving forward.
Correlation matrix of DNAm components (methylation patterns) and dimensions (MRPs). The mathematical representations of the MRPs are “dimensions”, and the gwDNAm patterns are “components”. Components 1–6 and 10 are strongly associated with confounders. We are unable to separate the biological variability of interest from that due to strong confounding in these components; thus, they will be dismissed. (As the sign of the correlation coefficient does not change the interpretation, it has been omitted for figure clarity)
In Step 2-A, we evaluate if gwDNAm patterns relate to child outcomes compared to the risk variables from which they were derived. For each outcome, we compared three models using R2 and MSE: Model 1—individual MRP-related variables, Model 2—MRP dimensions, and Model 3—MRP-based gwDNAm patterns.
Each model included confounders (see the “Methods” section—Confounder analysis) and shadow variables. Shadow variables are representations of predictor-outcome relations due to random chance (see Additional file 1: Tree-based analysis). Model 3 also includes cell count proportion estimates.
Figure 4 shows these models for waist circumference (internal z-score) at age 10. Early growth rates (i.e. before age 36 months) were the top-ranked variables in all three models. In Model 1, we observed that smoking-related variables were not ranked as relevant. This was consistent with traditional linear models (for example, the linear effect of maternal smoking for this outcome was β = − 0.09, p = 0.58). This was the general observation across various outcomes, except for two academic performance measures (see Additional file 2: Table S3). Model 1 always had a smaller sample size, largely due to missing self-reported maternal smoking data.
Comparison of models using DNAm patterns versus clinical variables using RF. Boxplot of importance scores (y-axis) of variables (x-axis) predicting waist circumference at age 10 using three different models (panels A–C). A Model 1 using individual MRP-related variables. B Model 2 using MRP dimensions from factor analysis. C Model 3 using gwDNAm patterns in cord blood. The x-axis includes additional control variables of estimated blood cell type proportions. Inset: Sample size and performance metrics for each model. Boxes appearing to the right of the grey vertical line (i.e. after the maximum randomised shadow variable) indicate relevant variables. The box and whiskers display the distribution of permutation values and cannot be used to tell statistical significance [31]. Blue—Dimension 1 (smoking in grandmother), orange—Dimension 3 (smoking in father + household + grandfather + mother), green—Dimension 4 (smoking in mother + birth weight), pink—Dimension 5 (smoking in household + mother + birth weight)
We observed that Dimension 4 and Component 7 (green boxes), as well as Dimension 1 and Component 9 (blue boxes), were selected in Models 2 and 3, respectively. These Dimension/Component pairs are correlated in Fig. 3. While their shared variability may explain the selection of these pairs, we also observed that Dimension 3 was selected in Model 2, but its correlate, Component 8, was not selected in Model 3. Component 18 was selected, but not Dimension 5. This could reflect differences in Dimension/Component correlations. However, it also suggests that the molecular patterns may hold variability that is distinct from the risk profile from which they were generated, thus changing the association to the same phenotype.
In Step 2-B, we compared the performances between Models 1, 2, and 3. The differences between the three models were minor (Fig. 4—inset), supporting the notion that the DNAm components model (Model 3) comparably captures the relation to subject outcomes.
We first review results from Model 3 using DNAm data collected at birth from cord blood. Component 9 was one of the most consistently relevant components among cardiometabolic outcomes (Additional file 2: Figs. S6-S9). This component was selected in the model of waist circumference at age 10 (Fig. 4C) and in models of blood pressure and lean mass at various ages. It was not related to weight at any age. Component 7 was related to lean mass, waist circumference, and weight at multiple ages. Component 18 demonstrated similar results for waist circumference, weight, blood pressure, and fat mass. Components 8 and 14 were related to a few outcomes at certain ages.
We observed that Components 9, 18, and 19 were related to parent-rated development at 6 months. Components 11, 13, and 19 were related to academic performance at various ages from about age 5 to 14. Component 19 was also related to intelligence assessed at 8 years (Additional file 2: Figures S10-S12, respectively). Neurocognitive outcomes generally had more missing data than cardiometabolic ones. For instance, 87% of subjects were missing data on intelligence measured at 4 years. Models for this outcome were very poor and did not generate stable predictors. Nearly all models selected paternal social status, which is consistent with other reports in this data set [38, 39]. This was also observed in Model 1. This consistency among certain variables is much like the behaviour of early growth variables in the cardiometabolic models.
Like all RF algorithms, Boruta does not accept missing values. Discarding incomplete observations has two important effects. It diminishes the sample size, which decreases the power to detect relevant variables. It also alters outlier and data noise effects on model specification. Thus, we performed a sensitivity analysis of RF models using DNAm data to compare three models: (1) Raw—using all variables and only complete (i.e. no missing data) observations; (2) Boruta—using only Boruta selected variables and only complete observations; and (3) Imputed—using all variables and all observations (i.e. missing data was imputed). As an example, Additional file 2: Table S1 shows how these three models compared using waist circumference at age 10. We observed little difference in error rates between the models among outcomes. Data for other outcomes is available upon request.
We examined whether DNAm patterns could be observed in children as they aged and in another cohort. Previous literature has shown that complex trait-related DNAm variability in cord blood has small effect sizes in the 1–2% range [40], a level that can easily be dwarfed by physiological, stochastic, and dataset-specific variability over time [41, 42]. We reasoned that if DNAm patterns are not biologically related to future phenotypes, then there would be little to no detectable relation between the two in different DNAm datasets. We tested the DNAm patterns trained on ARIES cord blood samples on DNAm data collected from (a) blood samples from ARIES subjects at ages 7 and 17 years and (b) cord blood samples from GenR.
The performance of models using DNAm data at older ages was similar compared to those using cord blood (Additional file 2: Figure S13 and Table S2). A subset of patterns persisted in their association with outcomes. For example, waist circumference was related to Component 18 in both cord blood and blood at age 17 years (Fig. 5). Blood pressure was related to Components 9 and 18 in blood samples at all three ages (Additional file 2: Figure S6). Similarly, Components 11, 13, and 19 were related to school performance at older ages (Additional file 2: Figure S10).
Summary of DNA methylation components relevant to waist circumference (z-score by sex) measured at ages 7, 9, 10, and 11 years (rows). Only components ranked as relevant at least once for this outcome are included. The legend shows the ages at which DNA methylation data was collected. The coloured text of component axis labels corresponds to the related dimension, as seen in Fig. 2. The results of Components 1–6 and 10 are not discussed as they demonstrate strong relations with confounders. The boxes with hashes indicate components that demonstrated the same relation to phenotype in DNAm data collected at more than one age. In other words, these hashed boxes indicate relations that persisted over time. Looking down the columns, we see that half the components that were related to waist circumference measured at a younger age would continue this association to age 11 years (i.e. coloured boxes are stacked vertically together). If the relation between DNAm components and future phenotype were due to chance or technical artefacts, this consistency as the phenotypic and molecular clocks ticked would be less likely. Results for other outcomes are in Additional file 2
Some associations with outcomes only appeared in DNAm in later childhood. For example, fat mass measured at ages 9, 11, and 13 years were all related to Component 9 but only in blood collected at age 7 (Additional file 2: Figure S7). Samples sizes for DNAm data in later childhood were larger as approximately 60 “new” subjects were added [24, 43] compared to the cord blood data. This gives us the advantage of having more testing data than training data and more power to detect associations.
We also point out that testing the relation to outcomes that precede DNAm data collection cannot support “prediction” in the same way as our findings from cord blood. Because most neurodevelopmental outcomes available for this study were measured in early childhood, only school performance has data collected prospectively or concurrently in relation to DNAm data collection. It is possible that a neurodevelopmental trait is reinforcing or generating the DNAm pattern, i.e. reversal causality. For example, the Denver developmental screen was last collected at 30 months of age, but the relation of Component 11 to these outcomes was only observed at ages 7 and 17.
We performed validation using 686 cord blood DNAm samples from GenR to predict body mass index (BMI) at age 6 years (Fig. 6). We compared this model to that from ARIES cord blood data and BMI at age 7 years. As in ARIES, early growth and components related to Dimension 4 (MRP representing smoking in the mother and household members and birth weight) were selected as relevant predictors in the GenR model (Fig. 6, data points in pink, Components 18 and 19). Components 7 and 11 (axis labels in bold in Fig. 6) were also selected. These components were relevant to several cardiometabolic outcomes in ARIES (see Fig. 3C for waist circumference and Additional file 2: Figure S6-S9 for weight, blood pressure, and fat and lean mass).
External validation of DNAm patterns. Boxplots of importance scores (y-axis) of variables (x-axis) predicting body mass index (BMI) in early childhood in the discovery cohort (ARIES) (A) versus independent replication cohort (Generation R) (B). Component 18 was selected in both cohorts. The replication cohort in B also ranked three other DNAm components that frequently showed relations to various other cardiometabolic outcomes (bold x-axis labels, Components 7, 11, and 19). Boxes appearing to the right of the grey vertical line (i.e. after the maximum randomised shadow variable) indicate relevant variables. The box and whiskers show the distribution of permutation values and cannot be used to tell statistical significance [31]. Purple—Dimension 2 (smoking in mother), green—Dimension 4 (smoking in mother + birth weight), pink—Dimension 5 (smoking in mother + household + birth weight). Inset: Sample size and performance metrics for each model. A Discovery Model from ARIES data using MRP DNAm patterns from cord blood to predict BMI (z-score by sex) at age 7 years. The x-axis includes the same control variables as in model 3 of Fig. 4C. B Validation Model from GenR data using MRP DNAm patterns in cord blood samples to predict BMI (z-score by sex) at age 6 years. The x-axis includes estimated cell type counts, sex, maternal education, and early growth (represented by BMI z-score measured between 13 and 17 months of age)
The GenR model had comparable performance metrics compared to other cardiometabolic outcome models using ARIES data (see Fig. 6 (inset) and Additional file 2: Table S2).
We took a more detailed look at the genomic localisation of gwDNAm patterns. PLS enables the empirical selection of DNAm sites that most consistently and strongly represent MRPs (described and schematically depicted in Additional file 1: Figure S3). This selection process adheres to our objective to view risk-related covariation in methylation as a coordinated regulatory event with interdependence between sites across the genome. This contrasts with individually testing associations between risk exposure and methylation (either as single or clusters of sites) in thousands of post hoc multiple hypothesis tests, such as performed in an epigenome-wide association study (EWAS).
We tested whether gwDNAm patterns co-localise with sites involved in the functional regulation of chromatin output. We used two features: (1) DNase I hypersensitivity sites (DHSs) and (2) promoter-anchored chromatin interaction (PAI) and chromatin loop sites. DNAm locations that spatially intersect with these two features are positioned in a 3D chromatin environment that is open and conformationally poised to interact with gene regulatory factors. Sites representative of gwDNAm patterns were enriched for DHSs and PAI/loop features (permutation p < 0.01 for both, Additional file 2: Figure S14). Figure 7 visualises the non-random organisation of DNAm sites (dark blue lines) within a gwDNAm pattern across the chromosomes in intersection with these 3D chromatin features (light blue and black lines).
Genomic organisation of DNAm sites representative of a DNAm pattern within the chromatin environment. We show Component 9 (related to Dimension 1—grandmaternal smoking) as an example. The perimeter ring shows the ideogram of the human autosomes with chromosome numbers. Unlike differentially methylated regions, which typically describe contiguous locations, genomic sites representing gwDNAm patterns (dark blue lines) are spread across a given chromosome and across a cell’s chromosomes. This is consistent with the finding that methylation related to complex traits is a genome-wide event. It is also in keeping with the interaction of non-contiguous domains of DNA by their physical proximation in 3D space through chromatin looping structures and promoter-anchored interaction sites (black lines). Regulatory mechanisms tend to occupy open chromatin areas. Such areas are marked by DNase I hypersensitivity sites (light blue lines). The three different coloured lines appear to populate together, much like how cities would concentrate the machinery required to “trade” information regarding foetal exposures across the genome, changing the global fate of the cell
To assess the functional relevance of EWAS candidates, most studies will test whether DNAm candidates are related to a gene product (usually from a coding gene that is linked to a certain mRNA, protein, or biological function like “inflammation”). Given that the 450K BeadChip was designed to interrogate DNAm at well-annotated genes, enrichment in such annotations would be unsurprising. We wondered whether the enrichments observed were simply due to the bias of the 450K BeadChip for certain genes, particularly genes that could demonstrate smoking-related changes but may have no biological effect on future health.
We challenged this query by testing whether genomic sites of a given gwDNAm pattern would still show greater than expected enrichment when directly compared to candidates identified by the largest-to-date meta-analysis of the association between sustained maternal smoking and cord blood DNAm (meta-EWAS) using the 450K BeadChip [44]. This study analysed associations across 13 different cohorts (n = 6685). The study team identified 2965 CpGs after correcting for multiple hypothesis testing, and 89% of these were associated with gene expression levels.
We used the XSTREME (meme-suite.org) analysis tool to evaluate enrichment in genomic sequences, called motifs, that exhibit affinity for binding to transcription factors. An enrichment is identified if the interrogated sites (in our case, sites representing a given gwDNAm pattern) can generate the same or more motifs of equal width and number of occurrences as the background sites (meaning meta-EWAS sites) based on log-likelihood ratios [45]. Most of the top 5 ranked motifs were novel (Additional file 2: Figure S15-S17). These novel motifs provide “an unbiased view of the in vivo DNA-binding propensities” [45] that represent possible novel or under-characterised regulators in referenced databases. Discarding the overlap with meta-EWAS candidates that were both large in number and closely associated with gene expression, sites representative of gwDNAm patterns were still localised to novel areas with an affinity for transcription regulation. In other words, the functional implications embedded within the physical distribution of DNAm patterns across the genome surpass what would be expected by chance or microarray-based bias.
We used locus overlap enrichment analysis (LOLA) and the curated LOLA database to determine whether gwDNAm patterns localised to tissue-specific chromatin marks [46]. gwDNAm patterns were enriched (q-value < 0.05) by chromatin marks specific to certain cell lineages, often with multiple enrichment clusters falling on the same or embryologically-related tissues (Fig. 8). For instance, Component 18 is dominated by high-ranking clusters specific to white blood cells. Component 9 is characterised by high-ranking clusters around two main panels: neuronal progenitor and iPS DF 19.11 (induced pluripotent stem cells derived from foreskin fibroblasts) cells, both arising from the neuroectodermal cell lineage. A similar enrichment in this cell lineage was observed in Component 19 (data not shown). In contrast, the meta-EWAS candidates harboured chromatin marks that were widely spread across cell lineages with no high-ranked clusters on specific cell lineages or marks from the same cell panels. These findings were consistent when using another tissue-specific molecular marker, DHSs [47] (Additional file 2: Figure S18).
Chromatin mark enrichment analysis using LOLA. X-axis: Enrichment rank where lower numbers indicate higher rank and thus stronger evidence of enrichment. Colours represent the source of DNAm candidates. Tissue/cell cultures demonstrating a specific chromatin mark are labelled on the y-axis. Cells sharing embryonic lineage are grouped together where applicable. Sites from a given DNAm pattern (i.e. same colour circles) tend to have two or more clusters with common lineages, as represented by circles falling on the same or nearby horizontal lines. In contrast, meta-EWAS candidates [44] represented by dark purple circles appear scattered across unrelated lineages. Granulocyte colony-stimulating factor, G-CSF; phorbol myristate acetate–ionomycin, PMA-I; human embryonic stem cell line, H1; induced pluripotent stem line derived from foreskin fibroblasts, iPS DF; human embryonic stem cell line, HUES
We first examined the overlap of our DNAm component candidates to the 28 maternal smoking-related CpG sites in cord blood identified in the same ARIES dataset by Richmond et al. [48]. Seven of our DNAm components overlapped with at least one of their hits (Additional file 2: Figure S20). Three of these components were related to confounders (Components 1 and 4 are related to sex, while Component 6 is related to sex and cell count). All overlaps had an associated target gene except for one (cg04598670).
Confounding from covariates like sex and cell count affect DNAm data distributions differently and can be cohort-specific [49, 50]. Most of the EWAS hits discovered by Richmond et al. overlap with EWAS hits in other cohorts [4, 51,52,53]. Therefore, we looked at the meta-EWAS to examine if this was specific to the ARIES dataset. Forty-eight percent (566/1183) of overlapping sites coincided with components related to sex and/or cell count confounders (Fig. 9).
Overlap of gwDNA methylation patterns with meta-EWAS candidates [44]. Histogram shows the number of EWAS candidates that match sites within DNAm components (patterns). The dark grey bars highlight seven DNAm components that we dismissed because they relate strongly with the confounders of sex and/or cell composition. Components related to confounders were also found to be frequently related to health outcomes. Among 1183 overlaps, 566 overlapped with these components (dark grey bars), 749 represented unique CpG sites, and 474 localised to unique genes. A large proportion of gwDNAm pattern representative sites “consistent” with this EWAS were related to Component 4. Recall that this component was related to subject sex and was the most strongly correlated component with the maternal smoking-related MRP, Dimension 2
The rise in NCD prevalence and the resources needed to treat their lifelong complications are draining to health systems and societies. Current detection methods require an adequate degree of cellular damage to indicate entry into the diseased state. The most cost-effective means of stemming this global crisis is detection that is generalisable to the whole population yet targets intervention before disease onset. We believe the key to achieving this feat is by considering the complex and context-specific nature of NCDs.
In this study, we adopted a view of the early origins of NCDs where (1) foetal risk exposure is a multidimensional and population-normalised entity and (2) gene regulation is a transformer of cell fate that requires numerous coordinated changes across the genome. We believe this is the first identification of patterns of DNAm underlying population-based risk profiles. We employed component-based analysis and model-based imputation to lessen the loss and/or distortion of information due to reliance on single points of data from subjects or variables alike. Importantly, this can reduce the impact of error and/or bias from subpopulation-specific characteristics [26, 54, 55].
As a proof-of-concept, we used MRPs that modelled the risks surrounding why and how mothers smoke while pregnant. We used this constellation of risks to reveal how early experiences influence the root of health during foetal development, branching into tissues and organs as phenotypic offshoots after birth, as hypothesised in DOHaD theory [56,57,58]. This may account for how NCDs associated with prenatal smoke exposure have a wide breadth of effects, such as respiratory, cardiometabolic, cognitive, and psychological morbidities [10, 11, 13, 15]. Pleiotropy is when a single gene contributes to more than one unrelated phenotypic trait, and it occurs frequently in NCDs [59, 60]. If an isolated genomic area can exhibit such pleiotropy, then this may also be true of epigenetic differences related to exposures [59, 61, 62]. Accordingly, we anticipated that gwDNAm patterns would be linked to both physical and neurodevelopmental outcomes.
Population-based gwDNAm patterns can help capture biological heterogeneity that comes from the interaction of genetic, environmental, and random effects. Decision tree-based analysis has the ability to account for these interactions, revealing relations that are difficult to detect using traditional linear models. This facilitates a more realistic view of interactive, robust, and prospective relations between gwDNAm patterns and future cardiometabolic and neurocognitive outcomes. We tested these models in DNAm data from mid- and late childhood and in an independent cohort. It was remarkable to observe the persistent association of DNAm to child phenotypes regardless of data influences such as developmental maturation, technical artefacts, and data set-specific variability [40].
Previous perinatal DNAm studies that have attempted a multifactorial approach focused on maternal psychosocial stress [63,64,65,66,67,68]. A study by Laubach et al. used a composite score of socioeconomic status using maternal factors collected in Project Viva, a multi-ethnic pregnancy cohort started in 1999 [65]. In an EWAS using the 450K BeadChip, they identified three CpGs in cord blood that were associated with low versus high socioeconomic scores. Only one was replicated at age 3, and none were replicated at age 7 years. We are unaware of any attempt to link these findings to phenotypes. A recent study by Provenzi et al. used the average score on six retrospective questions related to maternal stress due to COVID-19 infection [67]. These researchers only evaluated the methylation status of the SLC6A4 gene. They first found an association between COVID-19-related stress and methylation and, in a subsequent model, found the latter associated with only one out of three measured factors of infant behaviour. Most other studies use retrospective data or cannot capture foetal effects because of risk assessment or tissue collection at later ages (for a comprehensive review, see [69]).
Our aim to view each subject using a multidimensional and unique perspective could be described as “self-defeating” if judged by traditional statistical metrics. For instance, the PLS methodology seeks shared information between two datasets and does not maximise the variability explained. This directly undermines R2 and related metrics. Moreover, by avoiding the assumption that individuals fit into homogeneous categories, we cannot enhance the reported differential effect between groups. The non-linear and interactive effects detected by decision tree analysis are difficult to translate into straightforward results of causal, mediating, or dose–response effects, which are more familiar to clinicians, patients, and researchers [70].
DNAm data is also expensive and more invasive than routine history and physical measurements. However, we believe the value of gwDNAm patterns lies in how they may connect to real-life biological context. For example, the genome-wide distribution of these patterns intersects with various features that are integral to interactions with regulatory elements (e.g. locations associated with DHS, PAI, and transcription factor binding) and the 3D topology of chromatin (e.g. chromatin loop domains). This suggests that gwDNAm patterns have a spatial organisation linked to the functional units of cell fate decisions [71,72,73,74]. This is critical to operationalise one-dimensional measures of methylation into realistic reflections of the multidimensional and interactive nature of mechanisms underlying complex traits. Data supports that these chromatin features are key players in exposure-mediated shifts in gene expression and overall cell function [75]. This may also add to the potential forecasting ability of DNAm, given that the 3D re-organisation of chromatin can precede changes in gene expression [6, 73, 76].
It is possible that genotype-dependent methylation is responsible for our findings. However, we note that each gwDNAm pattern uses the magnitude and directionality of methylation at a given site relative to all other CpG sites across the genome to uncover consistent and coordinated patterns among individuals. Thus, DNAm sites within a given pattern are inherently related by design. Genotype-dependent methylation must act in magnitude and direction synchronously within gwDNAm patterns to give consistent false positive associations. If this biased the extraction of MRP-related gwDNAm patterns, then this same bias must also align itself to relate to outcomes across data sets spanning time, populations, and cardiometabolic and neurocognitive phenotypes. If that were the case, we reason that there should be far more consistent relations between differential methylation and child outcomes in the current literature [40].
CpGs representing a gwDNAm pattern are also conceptually different from differential DNAm sites identified in EWAS research because the latter have no innate relational organisation. This connectivity of sites within patterns reveals differences between patterns. We speculate these differences may underlie distinct processes such as cell- or stimuli-specific biological signalling. This was suggested by the degree of specificity in DNAm patterns, such as for certain tissues (Fig. 8) and for sources of variability due to blood cell type composition and sex (Fig. 9—dark bars). For example, eight meta-EWAS sites that annotate to CYP1A1 overlapped with Component 4 (which is related to sex and the maternal smoking-related MRP, Dimension 2) and with Components 9 and 11 (which were related to child outcomes). A similar scenario was observed for sites annotated to GFI1 and AHRR genes. One interpretation of these observations is that DNAm at these sites is confounded and should be discarded. However, we speculate that methylation (like for CYP1A1) is relevant to clinical outcomes depending on their interactions with genetic and epigenetic mechanisms at other sites that will vary in each individual. As such, there are instances where differential methylation at these same sites may be driven by “bystanders”, such as sex or cell composition that may covary or even interact with the exposure but do not lie at the biological root underpinning the phenotype. In other words, there may be specificity in the function of DNA methylation based on a broad scope of clinical and, thus, molecular contexts.
Previous literature has identified thousands of maternal smoking-related DNAm sites, many with theoretically plausible links to biological phenotypes. Compared to these (as represented by meta-EWAS data in our analysis), gwDNAm patterns were implicated in novel genomic sites (Fig. 9) and transcription networks (Additional file 2: Figures S15-S17) with a greater proportion of sites found in CpG poor or non-genic regions [77] (Additional file 3). Earlier studies have focused on candidates located around well-annotated protein-coding genes and biological pathways. This leads to a gene-centric bias, which is potentially harmful given that it may neglect lesser-known but potentially important mechanisms [78]. Mounting evidence suggests that the non-coding genome has a major role in human disease [79,80,81].
A disease-based bias currently exists, as the vast majority of gene annotations originate from repositories collected for cancers, rare diseases, or diseases mainly relevant to populations of European ancestry [82]. These potential biases are barriers to addressing the bench-to-bedside gap [5, 10, 78] as well as social disparities [83] in NCD research. As well, we are unaware of any epigenetic studies that have used a multidimensional, population-based measure of risk related to smoking to model foetal programming. Thus, our findings may promote the investigation of genomic regions previously neglected by this literature.
We anticipate that a context-based approach may complement traditional research findings by helping to explain why some DNAm sites that have received intense research focus (and garnered statistical “significance”) have provided no clinical traction in improving the management of NCDs [5, 78]. The intent of our study was not to “label” a child with his/her future outcomes. Like other NCD “biomarkers” in current literature (e.g. serotonin transporter gene polymorphisms (SLC6A4) and mental health disorders [84]), our work supports the notion that future outcomes are a result of many pathways where a biological factor may play different roles of varying importance depending on context.
Instead of interpreting these findings to suggest any degree of determinism conferred by life exposures, we believe they demonstrate the potential to uncover common molecular pathways underlying diverse vulnerabilities to risk. This capability will help the scientific and medical communities to foster resilience on a population scale. Further, our aim in exploring a context-based approach was to find a means to realistically model the intersection between genetic, environmental, and random chance effects that underlie maternal-foetal health. The uncovered models of gwDNAm patterns demonstrate reproducibility and specificity. As well, they probe novel interactions and functions among networks of DNAm sites that may offer insight into pathways to future health. We believe such efforts better harmonise science and bedside medicine, shifting the field towards context-based design and away from the use of discrete categories that invite stereotype-based labels.
We used DNAm data from blood cells. The extent of extrapolation of these findings to target tissues such as adipose and brain cells remains unknown, even though there are clear links between immune function in cardiometabolic and neurocognitive diseases [85, 86]. Though we attempted to attenuate subpopulation-based bias, it remains that the populations under study, microarray used, and various annotation resources are biased to represent primarily Western European-descent individuals. To evaluate generalisability, we will need to replicate and compare these results to those obtained from a mixed-descent population (after re-training and testing the methods). We used basic, open-source R language implementation tools in consideration of accessibility to all users and theoretic fit with our a priori hypotheses. More sophisticated methods (e.g. non-negative tensor factorisation or various artificial intelligence-based algorithms) exist that can better deal with the “high noise to low signal” data conditions and manage simultaneous learning and modelling between risk exposure data, DNAm data, and phenotypic outcomes. We hope this work will stimulate statistical methods in epigenetic and other omic studies that can better characterise and benchmark context-based representations of risks and outcomes. We were unable to compute patterns of change on a genome-wide scale despite having repeated measures of DNAm data due to the multiplicative computational cost of such a large data set. This is an area where techniques from fields like functional neuro- and cardiac-physiology [87] and biometry applications on smart devices [88] may be helpful in dealing with immense, repeated measures of data. We speculate such an advancement would improve model generalisability by increasing precision in characterising heterogeneity, enhancing the utility of limited human biological samples. Last, tree-based models can be difficult to interpret compared to main-effect models. As these methods continue to gain popularity in many fields outside of informatics, future models will have improved interpretability [89, 90].
Our findings are preliminary, but they join a growing body of research arguing for the exploration of coordinated patterns of molecular signals—signals that can funnel a wide diversity of risk profiles to a number of shared clinically relevant traits [91,92,93]. The gwDNAm patterns in this study provide a population-normalised score akin to how clinical tests have normative reference ranges. In addition to providing population-based context, the patterns demonstrate novel molecular context with remarkable colocation among genomic features critical to chromatin function. We believe our work represents a proof-of-concept for a context-based approach in the study of complex entities like the maternal-foetal dyad. If DNAm patterns discovered in blood can link population heterogeneity and molecular interactivity to future traits, this will enhance generalisability while individualising early risk management and avoiding invasive sampling of target tissues.
The data that support the findings of this study are available from the ALSPAC and Generation R executives, but restrictions apply to the availability of these data, which were used under licence for the current study and so are not publicly available. The ALSPAC study website (http://www.bristol.ac.uk/alspac/researchers/our-data/) contains details of all the data that are available through a fully searchable data dictionary and variable search tool. Data from Generation R are available upon reasonable request (generationr@erasmusmc.nl) and are subject to local and national regulations.
Avon Longitudinal Study of Parents and Children
Accessible Resource for Integrated Epigenomics Studies
Non-fatal, non-communicable common complex disease
Cytochrome P450 family 1 member A1 gene
Fat mass and obesity-associated gene
Growth factor independent 1 transcriptional repressor gene
Human embryonic stem cell line
Human embryonic stem cell line
Induced pluripotent stem line derived from foreskin fibroblasts
Meta-analysis of the association between sustained maternal smoking and cord blood DNAm, specifically the largest study to date conducted by (44).
Hypponen E, Smith GD, Power C. Effects of grandmothers’ smoking in pregnancy on birth weight: intergenerational cohort study. BMJ (Clinical research ed). 2003;327(7420):898.
Sugden K, Hannon EJ, Arseneault L, Belsky DW, Corcoran DL, Fisher HL, et al. Patterns of reliability: assessing the reproducibility and integrity of DNA methylation measurement. Patterns. 2020;1(2):100014.
Barker DJP. Maternal and fetal origins of cardiovascular disease. In: Fowkes FGR, editor. Epidemiology of Peripheral Vascular Disease. London: Springer; 1991. p. 247–54.
Knopik VS, Marceau K, Bidwell LC, Rolan E. Prenatal substance exposure and offspring development: does DNA methylation play a role? Neurotoxicol Teratol. 2019;71:50–63.
Article CAS PubMed Google Scholar
Akhabir L, Stringer R, Desai D, Mandhane PJ, Azad MB, Moraes TJ, et al. DNA methylation changes in cord blood and the developmental origins of health and disease - a systematic review and replication study. BMC Genomics. 2022;23(1):221.
Article CAS PubMed PubMed Central Google Scholar
Luo Y, Lu X, Xie H. Dynamic Alu methylation during normal development, aging, and tumorigenesis. BioMed Res Int. 2014;2014:784706.
Uhler C, Shivashankar GV. Regulation of genome organization and gene expression by nuclear mechanotransduction. Nat Rev Mol Cell Biol. 2017;18(12):717–27.
Article CAS PubMed Google Scholar
Doku DT, Acacio-Claro PJ, Koivusilta L, Rimpelä A. Social determinants of adolescent smoking over three generations. Scandinavian Journal of Public Health. 2020;48(6):646–56.
Erickson AC, Arbour LT. Heavy smoking during pregnancy as a marker for other risk factors of adverse birth outcomes: a population-based study in British Columbia, Canada. BMC Public Health. 2012;12(1):102.
Article PubMed PubMed Central Google Scholar
Cosin-Tomas M, Cilleros-Portet A, Aguilar-Lacasaña S, Fernandez-Jimenez N, Bustamante M. Prenatal maternal smoke, DNA methylation, and multi-omics of tissues and child health. Curr Environ Health Rep. 2022;9(3):502–12.
Article CAS PubMed PubMed Central Google Scholar
Li L, Peters H, Gama A, Carvalhal MI, Nogueira HG, Rosado-Marques V, et al. Maternal smoking in pregnancy association with childhood adiposity and blood pressure. Pediatr Obes. 2016;11(3):202–9.
Article CAS PubMed Google Scholar
Knopik VS. Maternal smoking during pregnancy and child outcomes: real or spurious effect? Dev Neuropsychol. 2009;34(1):1–36.
Article PubMed PubMed Central Google Scholar
Gilliland FD, Li Y-F, Peters JM. Effects of maternal smoking during pregnancy and environmental tobacco smoke on asthma and wheezing in children. Am J Respir Crit Care Med. 2001;163(2):429–36.
Article CAS PubMed Google Scholar
Biederman J, Monuteaux MC, Faraone SV, Mick E. Parsing the associations between prenatal exposure to nicotine and offspring psychopathology in a nonreferred sample. The Journal of adolescent health : official publication of the Society for Adolescent Medicine. 2009;45(2):142–8.
Leybovitz-Haleluya N, Wainstock T, Landau D, Sheiner E. Maternal smoking during pregnancy and the risk of pediatric cardiovascular diseases of the offspring: a population-based cohort study with up to 18-years of follow up. Reproductive toxicology (Elmsford, NY). 2018;78:69–74.
Forray A, Foster D. Substance use in the perinatal period. Curr Psychiatry Rep. 2015;17(11):91–5.
Article PubMed PubMed Central Google Scholar
Boyd A, Golding J, Macleod J, Lawlor DA, Fraser A, Henderson J, et al. Cohort profile: the ‘children of the 90s’—the index offspring of the Avon Longitudinal Study of Parents and Children. Int J Epidemiol. 2013;42(1):111–27.
Fraser A, Macdonald-Wallis C, Tilling K, Boyd A, Golding J, Davey Smith G, et al. Cohort profile: the Avon Longitudinal Study of Parents and Children: ALSPAC mothers cohort. Int J Epidemiol. 2013;42(1):97–110.
Cnattingius S. The epidemiology of smoking during pregnancy: smoking prevalence, maternal characteristics, and pregnancy outcomes. Nicotine & Tobacco Res. 2004;6(Suppl_2):S125–40.
Holloway AC, Cuu DQ, Morrison KM, Gerstein HC, Tarnopolsky MA. Transgenerational effects of fetal and neonatal exposure to nicotine. Endocrine. 2007;31(3):254–9.
Article CAS PubMed Google Scholar
Bruin JE, Gerstein HC, Holloway AC. Long-term consequences of fetal and neonatal nicotine exposure: a critical review. Toxicological sciences : an official journal of the Society of Toxicology. 2010;116(2):364–74.
Article CAS PubMed Google Scholar
Paternoster L, Howe LD, Tilling K, Weedon MN, Freathy RM, Frayling TM, et al. Adult height variants affect birth length and growth rate in children. Hum Mol Genet. 2011;20(20):4069–75.
Article CAS PubMed PubMed Central Google Scholar
Frankenburg WK, Dodds JB. The Denver developmental screening test. J Pediatr. 1967;71(2):181–91.
Article CAS PubMed Google Scholar
Relton CL, Gaunt T, McArdle W, Ho K, Duggirala A, Shihab H, et al. Data resource profile: accessible resource for integrated epigenomic studies (ARIES). Int J Epidemiol. 2015;44(4):1181–90.
Kooijman MN, Kruithof CJ, van Duijn CM, Duijts L, Franco OH, van Ijzendoorn MH, et al. The Generation R Study: design and cohort update 2017. Eur J Epidemiol.2016;31(12):1243–64.
Duda RO, Hart PE, Stork DG. Pattern Classification Wiley. New York: Wiley; 2001. p. 680.
Team RC. R: a language and environment for statistical computing. 2013.
Husson F, Josse J, Le S, Mazet J, Husson MF.Package 'FactoMineR.'An R package.2016;96:698.
Breiman L. Random Forests. Mach Learn. 2001;45(1):5–32.
Rai B. Feature selection and predictive modeling of housing data using random forest. World Academy of Science, Engineering and Technology, International Journal of Social, Behavioral, Educational, Economic, Business and Industrial Engineering. 2017;11(4):919–23.
Kursa MB, Rudnicki WR.Feature selection with the Boruta package.J StatSoft.2010;36(11):1–13.
Joubert BR, Haberg SE, Nilsen RM, Wang X, Vollset SE, Murphy SK, et al. 450K epigenome-wide scan identifies differential DNA methylation in newborns related to maternal smoking during pregnancy. Environ Health Perspect. 2012;120(10):1425–31.
Article CAS PubMed PubMed Central Google Scholar
Markunas CA, Xu Z, Harlid S, Wade PA, Lie RT, Taylor JA, et al. Identification of DNA methylation changes in newborns related to maternal smoking during pregnancy. Environ Health Perspect. 2014;122(10):1147–53.
Article PubMed PubMed Central Google Scholar
Reinius LE, Acevedo N, Joerink M, Pershagen G, Dahlén S-E, Greco D, et al. Differential DNA methylation in purified human blood cells: implications for cell lineage and studies on disease susceptibility. PLoS ONE. 2012;7(7): e41361.
Article CAS PubMed PubMed Central Google Scholar
Kuhn M. Building predictive models in R using the caret package. J Stat Softw. 2008;28(5):1–26.
Morris TJ, Butcher LM, Feber A, Teschendorff AE, Chakravarthy AR, Wojdacz TK, et al.ChAMP: 450k chip analysis methylation pipeline.Bioinformatics.2014;30(3):428–30.
Article CAS PubMed Google Scholar
Sharp GC, Lawlor DA, Richmond RC, Fraser A, Simpkin A, Suderman M, et al. Maternal pre-pregnancy BMI and gestational weight gain, offspring DNA methylation and later offspring adiposity: findings from the Avon Longitudinal Study of Parents and Children. Int J Epidemiol. 2015;44(4):1288–304.
Article PubMed PubMed Central Google Scholar
Macleod J, Hickman M, Bowen E, Alati R, Tilling K, Smith GD. Parental drug use, early adversities, later childhood problems and children’s use of tobacco and alcohol at age 10: birth cohort study. Addiction. 2008;103(10):1731–43.
Washbrook E, Propper C, Sayal K. Pre-school hyperactivity/attention problems and educational outcomes in adolescence: prospective longitudinal study. Br J Psychiatry. 2013;203(4):265–71.
Odintsova VV, Rebattu V, Hagenbeek FA, Pool R, Beck JJ, Ehli EA, et al. Predicting complex traits and exposures from polygenic scores and blood and buccal DNA methylation profiles. Front Psychiatry. 2021;12: 688464.
Article PubMed PubMed Central Google Scholar
Sala C, Di Lena P, Fernandes Durso D, Prodi A, Castellani G, Nardini C. Evaluation of pre-processing on the meta-analysis of DNA methylation data from the Illumina HumanMethylation450 BeadChip platform. PLoS ONE. 2020;15(3): e0229763.
Article CAS PubMed PubMed Central Google Scholar
Teschendorff AE, Relton CL. Statistical and integrative system-level analysis of DNA methylation data. Nat Rev Genet. 2018;19(3):129.
Article CAS PubMed Google Scholar
Northstone K, Lewcock M, Groom A, Boyd A, Macleod J, Timpson N, et al. The Avon Longitudinal Study of Parents and Children (ALSPAC): an update on the enrolled sample of index children in 2019. Wellcome Open Res. 2019;4:51.
Article PubMed PubMed Central Google Scholar
Joubert BR, Felix JF, Yousefi P, Bakulski KM, Just AC, Breton C, et al. DNA methylation in newborns and maternal smoking in pregnancy: genome-wide consortium meta-analysis. The American Journal of Human Genetics. 2016;98(4):680–96.
Article CAS PubMed Google Scholar
Grant CE, Bailey TL. XSTREME: comprehensive motif analysis of biological sequence datasets. BioRxiv. 2021.09. 02.458722.
Sheffield NC, Bock C. LOLA: enrichment analysis for genomic region sets and regulatory elements in R and Bioconductor. Bioinformatics. 2016;32(4):587–9.
Article CAS PubMed Google Scholar
Sheffield NC, Thurman RE, Song L, Safi A, Stamatoyannopoulos JA, Lenhard B, et al. Patterns of regulatory activity across diverse human cell types predict tissue identity, transcription factor binding, and long-range interactions. Genome Res. 2013;23(5):777–88.
Article CAS PubMed PubMed Central Google Scholar
Richmond RC, Simpkin AJ, Woodward G, Gaunt TR, Lyttleton O, McArdle WL, et al. Prenatal exposure to maternal smoking and offspring DNA methylation across the lifecourse: findings from the Avon Longitudinal Study of Parents and Children (ALSPAC). Hum Mol Genet. 2015;24(8):2201–17.
Article CAS PubMed Google Scholar
Bakulski KM, Feinberg JI, Andrews SV, Yang J, Brown S, L McKenney S, et al. DNA methylation of cord blood cell types: applications for mixed cell birth studies. Epigenetics. 2016;11(5):354–62.
Article PubMed PubMed Central Google Scholar
Suderman M, Simpkin A, Sharp G, Gaunt T, Lyttleton O, McArdle W, et al. Sex-associated autosomal DNA methylation differences are wide-spread and stable throughout childhood. Biorxiv. 2017:118265.
Lee KWK, Richmond R, Hu P, French L, Shin J, Bourdon C, et al. Prenatal exposure to maternal cigarette smoking and DNA methylation: epigenome-wide association in a discovery sample of adolescents and replication in an independent cohort at birth through 17 years of age. Environ Health Perspect. 2014;123(2):193–9.
Article PubMed PubMed Central Google Scholar
Wiklund P, Karhunen V, Richmond RC, Parmar P, Rodriguez A, De Silva M, et al. DNA methylation links prenatal smoking exposure to later life health outcomes in offspring. Clin Epigenetics. 2019;11(1):97.
Article PubMed PubMed Central Google Scholar
Kupers LK, Xu X, Jankipersadsing SA, Vaez A, la Bastide-van GS, Scholtens S, et al. DNA methylation mediates the effect of maternal smoking during pregnancy on birthweight of the offspring. Int J Epidemiol. 2015;44(4):1224–37.
Article PubMed PubMed Central Google Scholar
van Walraven C, Hart RG. Leave ’em alone - why continuous variables should be analyzed as such. Neuroepidemiology. 2008;30(3):138–9.
Rosenbaum PR, Rubin DB. Reducing bias in observational studies using subclassification on the propensity score. J Am Stat Assoc. 1984;79(387):516–24.
Drake AJ, Walker BR. The intergenerational effects of fetal programming: non-genomic mechanisms for the inheritance of low birth weight and cardiovascular risk. J Endocrinol. 2004;180(1):1–16.
Article CAS PubMed Google Scholar
Buck JM, Sanders KN, Wageman CR, Knopik VS, Stitzel JA, O’Neill HC. Developmental nicotine exposure precipitates multigenerational maternal transmission of nicotine preference and ADHD-like behavioral, rhythmometric, neuropharmacological, and epigenetic anomalies in adolescent mice. Neuropharmacology. 2019;149:66–82.
Article CAS PubMed PubMed Central Google Scholar
Serpeloni F, Radtke K, de Assis SG, Henning F, Nätt D, Elbert T. Grandmaternal stress during pregnancy and DNA methylation of the third generation: an epigenome-wide association study. Transl Psychiatry. 2017;7(8): e1202.
Article CAS PubMed PubMed Central Google Scholar
Yang C, Li C, Wang Q, Chung D, Zhao H. Implications of pleiotropy: challenges and opportunities for mining Big Data in biomedicine. Front Genet. 2015;6:229.
Article CAS PubMed PubMed Central Google Scholar
Visscher PM, Brown MA, McCarthy MI, Yang J. Five years of GWAS discovery. Am J Hum Genet. 2012;90(1):7–24.
Article CAS PubMed PubMed Central Google Scholar
Vattikuti S, Guo J, Chow CC. Heritability and genetic correlations explained by common SNPs for metabolic syndrome traits. PLoS Genet. 2012;8(3): e1002637.
Article CAS PubMed PubMed Central Google Scholar
Shah S, Bonder MJ, Marioni RE, Zhu Z, McRae AF, Zhernakova A, et al. Improving phenotypic prediction by combining genetic and epigenetic associations. Am J Hum Genet. 2015;97(1):75–85.
Article CAS PubMed PubMed Central Google Scholar
Cerutti J, Lussier AA, Zhu Y, Liu J, Dunn EC. Associations between indicators of socioeconomic position and DNA methylation: a scoping review. Clin Epigenetics. 2021;13(1):221.
Article CAS PubMed PubMed Central Google Scholar
Azar N, Booij L. DNA methylation as a mediator in the association between prenatal maternal stress and child mental health outcomes: Current state of knowledge. J Affect Disord. 2022;319:142–63.
Article CAS PubMed Google Scholar
Laubach ZM, Perng W, Cardenas A, Rifas-Shiman SL, Oken E, DeMeo D, et al.Socioeconomic status and DNA methylation from birth through mid-childhood: a prospective study in Project Viva.Epigenomics.2019;11(12):1413–27.
Article CAS PubMed PubMed Central Google Scholar
Santos HP, Bhattacharya A, Martin EM, Addo K, Psioda M, Smeester L, et al. Epigenome-wide DNA methylation in placentas from preterm infants: association with maternal socioeconomic status. Epigenetics. 2019;14(8):751–65.
Article PubMed PubMed Central Google Scholar
Provenzi L, Grumi S, Altieri L, Bensi G, Bertazzoli E, Biasucci G, et al.Prenatal maternal stress during the COVID-19 pandemic and infant regulatory capacity at 3 months: a longitudinal study.Dev Psychopathol.2023;35(1):35–43.
Polinski KJ, Putnick DL, Robinson SL, Schliep KC, Silver RM, Guan W, et al. Periconception and prenatal exposure to maternal perceived stress and cord blood DNA methylation. Epigenetics Insights. 2022;15:25168657221082044.
Article PubMed PubMed Central Google Scholar
Schrott R, Song A, Ladd-Acosta C. Epigenetics as a biomarker for early-life environmental exposure. Curr Environ Health Rep. 2022;9(4):604–24.
Article CAS PubMed Google Scholar
Gilpin LH, Bau D, Yuan BZ, Bajwa A, Specter M, Kagal L. Explaining explanations: an overview of interpretability of machine learning. 2018 IEEE 5th International Conference on Data Science and Advanced Analytics (DSAA). Turin; 2018. p. 80–9.
Li G, Liu Y, Zhang Y, Kubo N, Yu M, Fang R, et al. Joint profiling of DNA methylation and chromatin architecture in single cells. Nat Methods. 2019;16(10):991–3.
Article CAS PubMed PubMed Central Google Scholar
Xue Y, Yang Y, Tian H, Quan H, Liu S, Zhang L, et al. Computational characterization of domain-segregated 3D chromatin structure and segmented DNA methylation status in carcinogenesis. Mol Oncol. 2022;16(3):699–716.
Article CAS PubMed Google Scholar
Liu S, Zhang L, Quan H, Tian H, Meng L, Yang L, et al. From 1D sequence to 3D chromatin dynamics and cellular functions: a phase separation perspective. Nucleic Acids Res. 2018;46(18):9367–83.
Article CAS PubMed PubMed Central Google Scholar
Zhang L, Xie WJ, Liu S, Meng L, Gu C, Gao YQ. DNA methylation landscape reflects the spatial organization of chromatin in different cells. Biophys J. 2017;113(7):1395–404.
Article CAS PubMed PubMed Central Google Scholar
Schvartzman JM, Thompson CB, Finley LWS. Metabolic regulation of chromatin modifications and gene expression. J Cell Biol. 2018;217(7):2247–59.
Article CAS PubMed PubMed Central Google Scholar
Xu H, Zhang S, Yi X, Plewczynski D, Li MJ. Exploring 3D chromatin contacts in gene regulation: The evolution of approaches for the identification of functional enhancer-promoter interaction. Comput Struct Biotechnol J. 2020;18:58–570.
Bacalini MG, Boattini A, Gentilini D, Giampieri E, Pirazzini C, Giuliani C, et al. A meta-analysis on age-associated changes in blood DNA methylation: results from an original analysis pipeline for Infinium 450k data. Aging. 2015;7(2):97–109.
Article CAS PubMed PubMed Central Google Scholar
Haynes WA, Tomczak A, Khatri P. Gene annotation bias impedes biomedical research.Sci Rep.2018;8(1):1
Article PubMed PubMed Central Google Scholar
Dhanasekaran K, Kumari S, Kanduri C. Noncoding RNAs in chromatin organization and transcription regulation: an epigenetic view. Subcell Biochem. 2013;61:343–72.
Article CAS PubMed Google Scholar
Tak YG, Farnham PJ. Making sense of GWAS: using epigenomics and genome engineering to understand the functional relevance of SNPs in non-coding regions of the human genome. Epigenetics & chromatin. 2015;8:57–4 eCollection 2015.
Friedman RC, Farh KK, Burge CB, Bartel DP. Most mammalian mRNAs are conserved targets of microRNAs. Genome Res. 2009;19(1):92–105.
Article CAS PubMed PubMed Central Google Scholar
Ju D, Hui D, Hammond DA, Wonkam A, Tishkoff SA. Importance of including non-European populations in large human genetic studies to enhance precision medicine. Annu Rev Biomed Data Sci. 2022;5(1):321–39.
Article PubMed PubMed Central Google Scholar
Ragland DR. Dichotomizing continuous outcome variables: dependence of the magnitude of association and statistical power on the cutpoint. Epidemiology. 1992;3(5):434–40.
Article CAS PubMed Google Scholar
Homberg JR, Lesch KP. Looking on the bright side of serotonin transporter gene variation. Biol Psychiatry. 2011;69(6):513–9.
Article CAS PubMed Google Scholar
Stunnenberg HG, Abrignani S, Adams D, de Almeida M, Altucci L, Amin V, et al. The International Human Epigenome Consortium: a blueprint for scientific collaboration and discovery. Cell. 2016;167(5):1145–9.
Article CAS PubMed Google Scholar
Roadmap EC, Kundaje A, Meuleman W, Ernst J, Bilenky M, Yen A, et al. Integrative analysis of 111 reference human epigenomes. Nature. 2015;518:317.
Van Eyndhoven S. Tensor-based Blind Source Separation for Structured EEG-fMRI Data Fusion. 2020.
Mekruksavanich S, Jitpattanakul A. Biometric user identification based on human activity recognition using wearable sensors: an experiment using deep learning models. Electronics. 2021;10(3):308.
Nohara Y, Matsumoto K, Soejima H, Nakashima N. Explanation of machine learning models using shapley additive explanation and application for real data in hospital. Comput Methods Programs Biomed. 2022;214: 106584.
Rauschert S, Melton PE, Heiskala A, Karhunen V, Burdge G, Craig JM, et al. Machine learning-based DNA methylation score for fetal exposure to maternal smoking: development and validation in samples collected from adolescents and adults. Environ Health Perspect. 2020;128(9): 097003.
Article PubMed PubMed Central Google Scholar
Uher R. The implications of gene-environment interactions in depression: will cause inform cure? Mol Psychiatry. 2008;13(12):1070–8.
Article CAS PubMed Google Scholar
Xu Y, Wu M, Ma S. Multidimensional molecular measurements–environment interaction analysis for disease outcomes. Biometrics. 2022;78(4):1542–54.
Mordaunt CE, Mouat JS, Schmidt RJ, LaSalle JM. Comethyl: a network-based methylome approach to investigate the multivariate nature of health and disease. Briefings in bioinformatics. 2022;23(2):bbab554.
Article PubMed PubMed Central Google Scholar
Villar J, Cheikh Ismail L, Victora CG, Ohuma EO, Bertino E, Altman DG, et al. International standards for newborn weight, length, and head circumference by gestational age and sex: the Newborn Cross-Sectional Study of the INTERGROWTH-21st Project. Lancet (London, England). 2014;384(9946):857–68.
Selvin S. Statistical power and sample-size calculations. Statistical analyses of epidemiologic data. 1996;2:95–100.
Pidsley R, Y Wong CC, Volta M, Lunnon K, Mill J, Schalkwyk LC. A data-driven approach to preprocessing Illumina 450K methylation array data. BMC genomics. 2013;14:293.
Article CAS PubMed PubMed Central Google Scholar
Touleimat N, Tost J. Complete pipeline for Infinium((R)) Human Methylation 450K BeadChip data processing using subset quantile normalization for accurate DNA methylation estimation. Epigenomics. 2012;4(3):325–41.
Article CAS PubMed Google Scholar
Chen YA, Lemire M, Choufani S, Butcher DT, Grafodatskaya D, Zanke BW, et al. Discovery of cross-reactive probes and polymorphic CpGs in the Illumina Infinium HumanMethylation450 microarray. Epigenetics : official journal of the DNA Methylation Society. 2013;8(2):203–9.
Peters TJ, Buckley MJ, Statham AL, Pidsley R, Samaras K, Lord RV, et al. De novo identification of differentially methylated regions in the human genome. Epigenetics Chromatin. 2015;8(1):6.
Article PubMed PubMed Central Google Scholar
Zhi D, Aslibekyan S, Irvin MR, Claas SA, Borecki IB, Ordovas JM, et al. SNPs located at CpG sites modulate genome-epigenome interaction. Epigenetics. 2013;8(8):802–6.
Article CAS PubMed PubMed Central Google Scholar
Zhou W, Laird PW, Shen H. Comprehensive characterization, annotation and innovative use of Infinium DNA methylation BeadChip probes. Nucleic Acids Res. 2017;45(4): e22.
Zhou D, Li Z, Yu D, Wan L, Zhu Y, Lai M, et al. Polymorphisms involving gain or loss of CpG sites are significantly enriched in trait-associated SNPs. Oncotarget. 2015;6(37):39995–40004.
Article PubMed PubMed Central Google Scholar
Gutierrez-Arcelus M, Lappalainen T, Montgomery SB, Buil A, Ongen H, Yurovsky A, et al. Passive and active DNA methylation and the interplay with genetic variation in gene regulation. elife. 2013;2:e00523.
Article PubMed PubMed Central Google Scholar
Lappalainen T, Greally JM. Associating cellular epigenetic models with human phenotypes. Nat Rev Genet. 2017;18(7):441.
Article CAS PubMed Google Scholar
Gaunt TR, Shihab HA, Hemani G, Min JL, Woodward G, Lyttleton O, et al. Systematic identification of genetic influences on methylation across the human life course. Genome Biol. 2016;17(1):1–14.
Teh AL, Pan H, Chen L, Ong M-L, Dogra S, Wong J, et al. The effect of genotype and in utero environment on interindividual variation in neonate DNA methylomes. Genome Res. 2014;24(7):1064–74.
Article CAS PubMed PubMed Central Google Scholar
Barfield RT, Almli LM, Kilaru V, Smith AK, Mercer KB, Duncan R, et al. Accounting for population stratification in DNA methylation studies. Genet Epidemiol. 2014;38(3):231–41.
Article PubMed PubMed Central Google Scholar
Shang L, Zhao W, Wang YZ, Li Z, Choi JJ, Kho M, et al. meQTL mapping in the GENOA study reveals genetic determinants of DNA methylation in African Americans. Nat Commun. 2023;14(1):2711.
Article CAS PubMed PubMed Central Google Scholar
Gunasekara CJ, MacKay H, Scott CA, Li S, Laritsky E, Baker MS, et al. Systemic interindividual epigenetic variation in humans is associated with transposable elements and under strong genetic control. Genome Biol. 2023;24(1):2.
Article CAS PubMed PubMed Central Google Scholar
Edgar RD, Jones MJ, Robinson WP, Kobor MS. An empirically driven data reduction method on the human 450K methylation array to remove tissue specific non-variable CpGs. Clin Epigenetics. 2017;9(1):1–8.
Esposito EA, Jones MJ, Doom JR, MacIsaac JL, Gunnar MR, Kobor MS. Differential DNA methylation in peripheral blood mononuclear cells in adolescents exposed to significant early but not later childhood adversity. Dev Psychopathol. 2016;28(4 Pt 2):1385.
Article PubMed PubMed Central Google Scholar
Derakhshan M, Kessler NJ, Ishida M, Demetriou C, Brucato N, Moore GE, et al. Tissue- and ethnicity-independent hypervariable DNA methylation states show evidence of establishment in the early human embryo. Nucleic Acids Res. 2022;50(12):6735–52.
Article CAS PubMed PubMed Central Google Scholar
Renard E, Absil PA. Comparison of location-scale and matrix factorization batch effect removal methods on gene expression datasets. 2017 IEEE International Conference on Bioinformatics and Biomedicine (BIBM), Kansas City; 2017. p. 1530–7.
Min JL, Hemani G, Davey Smith G, Relton C, Suderman M. Meffil: efficient normalization and analysis of very large DNA methylation datasets. Bioinformatics. 2018;34(23):3983–9.
Article CAS PubMed PubMed Central Google Scholar
Timms JA, Relton CL, Sharp GC, Rankin J, Strathdee G, McKay JA. Exploring a potential mechanistic role of DNA methylation in the relationship between in utero and post-natal environmental exposures and risk of childhood acute lymphoblastic leukaemia. Int J Cancer. 2019;145(11):2933–43.
Article CAS PubMed PubMed Central Google Scholar
Houseman EA, Molitor J, Marsit CJ. Reference-free cell mixture adjustments in analysis of DNA methylation data. Bioinformatics (Oxford, England). 2014;30(10):1431–9.
Rahmani E, Zaitlen N, Baran Y, Eng C, Hu D, Galanter J, et al. Sparse PCA corrects for cell type heterogeneity in epigenome-wide association studies. Nat Methods. 2016;13(5):443–5.
Article CAS PubMed PubMed Central Google Scholar
Bonder MJ , Kasela S , Kals M , Tamm R , Lokk K , Barragan I , et al.Genetic and epigenetic regulation of gene expression in fetal and adult human livers.BMC genomics.2014;15:8
Article PubMed PubMed Central Google Scholar
Aryee MJ, Jaffe AE, Corrada-Bravo H, Ladd-Acosta C, Feinberg AP, Hansen KD, et al. Minfi: a flexible and comprehensive Bioconductor package for the analysis of Infinium DNA methylation microarrays. Bioinformatics. 2014;30(10):1363–9.
Article CAS PubMed PubMed Central Google Scholar
Sharp GC, Arathimos R, Reese SE, Page CM, Felix J, Kupers LK, et al. Maternal alcohol consumption and offspring DNA methylation: findings from six general population-based birth cohorts. Epigenomics. 2018;10(1):27–42.
Article CAS PubMed Google Scholar
Kassambara A. Practical guide to principal component methods in R: PCA, M (CA), FAMD, MFA, HCPC, factoextra. Sthda; 2017.
Mevik BH, Cederkvist HR. Mean squared error of prediction (MSEP) estimates for principal component regression (PCR) and partial least squares regression (PLSR). J Chemom. 2004;18(9):422–9.
Cao-Lei L, Elgbeili G, Szyf M, Laplante DP, King S. Differential genome-wide DNA methylation patterns in childhood obesity. BMC Res Notes. 2019;12(1):174.
Article PubMed PubMed Central Google Scholar
Liquet B, de Micheaux PL, Hejblum BP, Thiebaut R. Group and sparse group partial least square approaches applied in genomics context. Bioinformatics (Oxford, England). 2016;32(1):35–42.
Gonzalez I, Cao KA, Davis MJ, Dejean S. Visualising associations between paired “omics” data sets. BioData mining. 2012;5(1):19.
Article PubMed PubMed Central Google Scholar
Hore V, Viñuela A, Buil A, Knight J, McCarthy MI, Small K, et al. Tensor decomposition for multiple-tissue gene expression experiments. Nat Genet. 2016;48:1094.
Article CAS PubMed PubMed Central Google Scholar
Karimpour-Fard A, Epperson LE, Hunter LE. A survey of computational tools for downstream analysis of proteomic and other omic datasets. Hum Genomics. 2015;9(1):28.
Article PubMed PubMed Central Google Scholar
Degenhardt F, Seifert S, Szymczak S. Evaluation of variable selection methods for random forests and omics data sets. Brief Bioinform. 2019;20(2):492–503.
Kursa MB. Robustness of Random Forest-based gene selection methods. BMC Bioinformatics. 2014;15(1):8.
Article PubMed PubMed Central Google Scholar
Lunetta KL, Hayward LB, Segal J, Van Eerdewegh P. Screening large-scale association study data: exploiting interactions using random forests. BMC Genet. 2004;5(1):32.
Article PubMed PubMed Central Google Scholar
Winham SJ, Colby CL, Freimuth RR, Wang X, De Andrade M, Huebner M, et al. SNP interaction detection with random forests in high-dimensional genetic data. BMC Bioinformatics. 2012;13(1):164.
Article PubMed PubMed Central Google Scholar
Diaz-Uriarte R, Alvarez de Andres S. Gene selection and classification of microarray data using random forest. BMC bioinformatics. 2006;7:3.
Article PubMed PubMed Central Google Scholar
Li J, Tran M, Siwabessy J. Selecting Optimal Random Forest Predictive Models: A Case Study on Predicting the Spatial Distribution of Seabed Hardness. PLoS ONE. 2016;11(2):e0149089.
Article PubMed PubMed Central Google Scholar
van der Meer D, Hoekstra PJ, Van Donkelaar M, Bralten J, Oosterlaan J, Heslenfeld D, et al. Predicting attention-deficit/hyperactivity disorder severity from psychosocial stress and stress-response genes: a random forest regression approach. Transl Psychiatry. 2017;7(6):e1145.
Article PubMed PubMed Central Google Scholar
Ishwaran H, Kogalur UB, Kogalur MUB.Package 'randomForestSRC'.Version.2023.
Schafer JL, Graham JW. Missing data: our view of the state of the art. Psychol Methods. 2002;7(2):147–77.
Clark EM, Ness A, Tobias JH. Social Position Affects Bone Mass in Childhood Through Opposing Actions on Height and Weight. J Bone Miner Res. 2005;20(12):2082–9.
Alfano R, Guida F, Galobardes B, Chadeau-Hyam M, Delpierre C, Ghantous A, et al. Socioeconomic position during pregnancy and DNA methylation signatures at three stages across early life: epigenome-wide association studies in the ALSPAC birth cohort. Int J Epidemiol. 2019;48(1):30–44.
Howe LD, Tilling K, Galobardes B, Smith GD, Ness AR, Lawlor DA. Socioeconomic disparities in trajectories of adiposity across childhood. International journal of pediatric obesity : IJPO : an official journal of the International Association for the Study of Obesity. 2011;6(2–2):144.
Howe LD, Tilling K, Galobardes B, Smith GD, Gunnell D, Lawlor DA. Socioeconomic differences in childhood growth trajectories: at what age do height inequalities emerge? J Epidemiol Community Health. 2012;66(2):143–8.
Agha G, Hajj H, Rifas-Shiman SL, Just AC, Hivert M-F, Burris HH, et al. Birth weight-for-gestational age is associated with DNA methylation at birth and in childhood. Clin Epigenetics. 2016;8(1):118.
Article PubMed PubMed Central Google Scholar
Gel B, Díez-Villanueva A, Serra E, Buschbeck M, Peinado MA, Malinverni R. regioneR: an R/Bioconductor package for the association analysis of genomic regions based on permutation tests. Bioinformatics. 2015;32(2):289–91.
Article PubMed PubMed Central Google Scholar
Iles-Caven Y, Golding J, Gregory S, Emond A, Taylor CM. Data relating to early child development in the Avon Longitudinal Study of Parents and Children (ALSPAC), their relationship with prenatal blood mercury and stratification by fish consumption. Data Brief. 2016;9:112–22.
Article PubMed PubMed Central Google Scholar
Booth JN, Tomporowski PD, Boyle JME, Ness AR, Joinson C, Leary SD, et al. Obesity impairs academic attainment in adolescence: findings from ALSPAC, a UK cohort. Int J Obes. 2014;38(10):1335–42.
Taylor CM, Kordas K, Golding J, Emond AM. Data relating to prenatal lead exposure and child IQ at 4 and 8 years old in the Avon Longitudinal Study of Parents and Children. Neurotoxicology. 2017;62:224–30.
Article CAS PubMed PubMed Central Google Scholar
Ecker S, Chen L, Pancaldi V, Bagger FO, Fernández JM, Carrillo de Santa Pau E, et al. Genome-wide analysis of differential transcriptional and epigenetic variability across human immune cell types. Genome Biol. 2017;18(1):18.
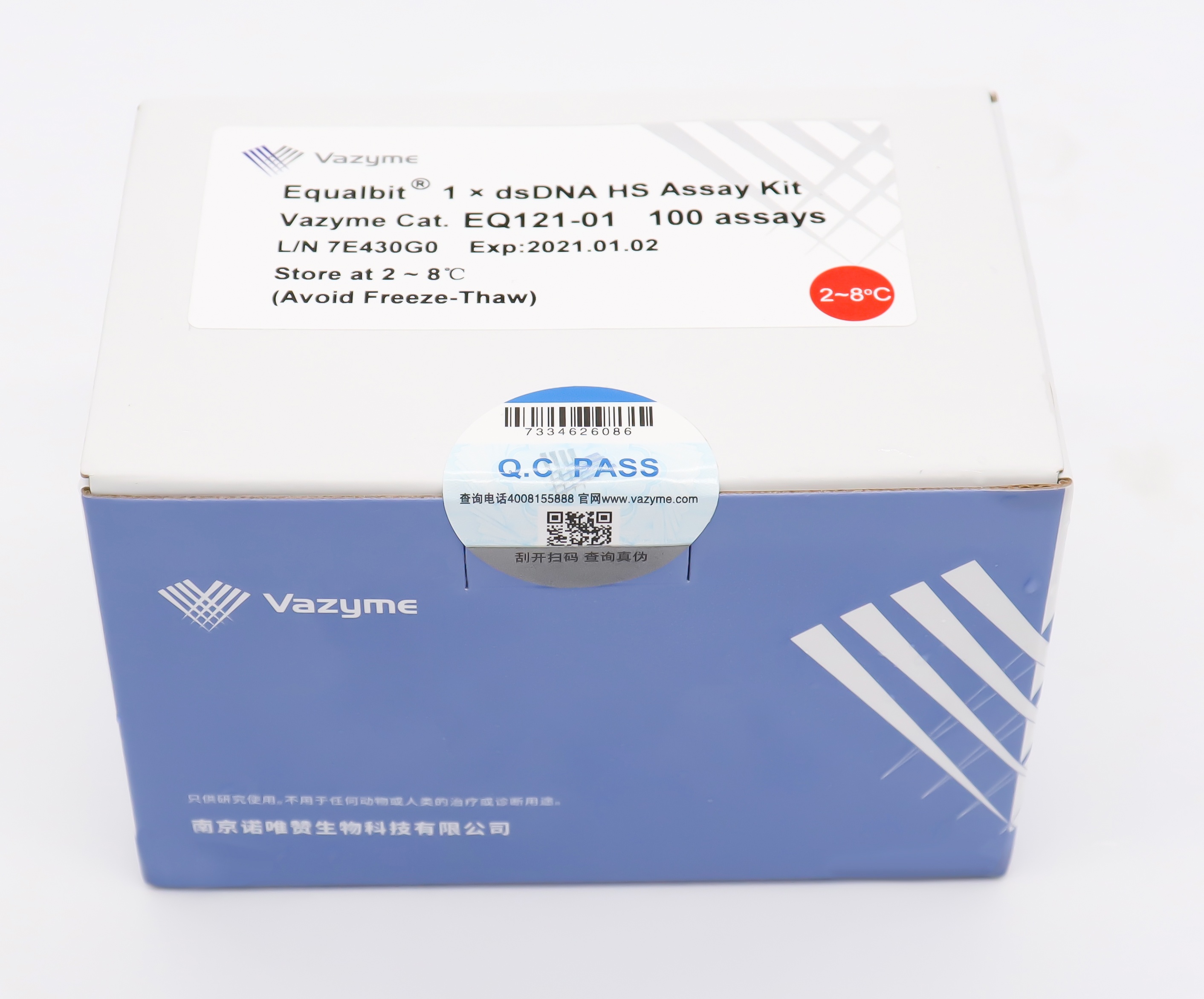
Nucleic Acid Electrophoresis Article PubMed PubMed Central